Invited Talks
A common model of AI suggests that there is a single measure of intelligence, often called AGI, and that AI systems are agents who can possess more or less of this intelligence. Cognitive science, in contrast, suggests that there are multiple forms of intelligence and that these intelligences trade-off against each other and have a distinctive developmental profile. The adult ability to accomplish goals and maximize utilities is often seen as the quintessential form of intelligence. However, this ability to exploit is in tension with the ability to explore. Children are particularly adept at exploration, though at the cost of competent action and decision-making. Human intelligence also relies heavily on cultural transmission, passing on information from one generation to the next, and children are also particularly adept at such learning.Thinking about exploration and transmission can change our approach to AI systems. Large language models and similar systems are best understood as cultural technologies, like writing, pictures and print, that enable information transmission. In contrast, our empirical work suggests that RL systems employing an intrinsic objective of empowerment gain can help capture the exploration we see in children.
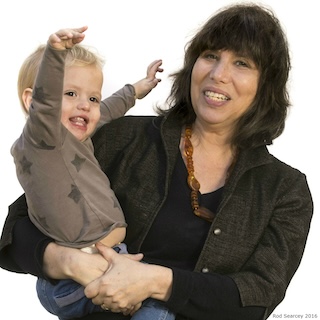
Alison Gopnik
Alison Gopnik is a professor of psychology and affiliate professor of philosophy at the University of California at Berkeley. She received her BA from McGill University and her PhD. from Oxford University. She is an internationally recognized leader in the study of children’s learning and development and was one of the founders of the field of “theory of mind”, an originator of the “theory theory” of children’s development and more recently introduced the idea that probabilistic models and Bayesian inference could be applied to children’s learning. She has held a Center for Advanced Studies in the Behavioral Sciences Fellowship, the Moore Distinguished Scholar fellowship at the California Institute of Technology, the All Souls College Distinguished Visiting Fellowship at Oxford, and King’s College Distinguished Visiting Fellowship at Cambridge. She is an elected member of the Society of Experimental Psychologists and the American Academy of Arts and Sciences and a fellow of the Cognitive Science Society. She has been continuously supported by the NSF and was PI on a 2.5 million dollar interdisciplinary collaborative grant on causal learning from the McDonnell Foundation.
She is the author or coauthor of over 100 journal articles and several books including “Words, thoughts and theories” MIT Press, 1997, and the bestselling and critically acclaimed popular books “The Scientist in the Crib” William Morrow, 1999, and “The Philosophical Baby; What children’s minds tell us about love, truth and the meaning of life”, which won the Cognitive Development Society Best Book Prize in 2011, and The Gardener and the Carpenter, Farrar, Strauss and Giroux, 2009, 2016. She has also written widely about cognitive science and psychology for Science, The New York Times, Scientific American, The New Yorker, The Times Literary Supplement, The New York Review of Books, New Scientist and Slate, among others. Her TED talk on her work has been viewed more than 2.8 million times. And she has frequently appeared on TV and radio including “The Charlie Rose Show” and “The Colbert Report”. Since 2013 she has written the Mind and Matter column for the Wall Street Journal.
Technological change typically occurs in three phases: basic research, scale-up, and industrial application, each with a different degree of methodological diversity—high, low, and medium, respectively. Historically, breakthroughs such as the steam engine and the Haber-Bosch process exemplify these phases and have had a profound impact on society. A similar pattern can be observed in the development of modern artificial intelligence (AI). In the scale-up phase of AI, large language models (LLMs) have emerged as the most prominent example. While LLMs can be seen as highly sophisticated knowledge representation techniques, they have not fundamentally advanced AI itself. The upscaling phase of AI was dominated by the transformer architecture. More recently, other architectures, such as state-space models and recurrent neural networks, have also been scaled up. For example, Long Short-Term Memory (LSTM) networks have been scaled up to xLSTM, which in many cases outperform transformers. We are now transitioning into the third phase: industrial AI. In this phase, we are adapting AI methods to real-world applications in robotics, life and earth sciences, engineering, or large-scale simulations that can be dramatically accelerated by AI methods. As we continue to develop these industrial AI methods, we expect to see an increase in methodological diversity, allowing us to overcome what has been called the "bitter lesson" of scaling up.
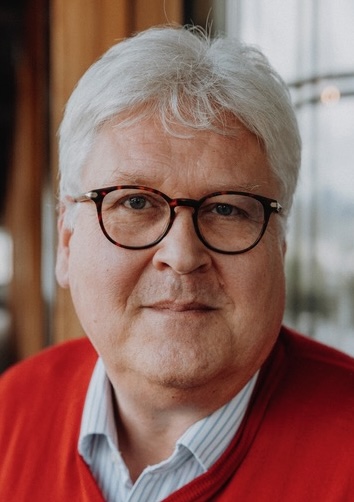
Sepp Hochreiter
Sepp Hochreiter heads the Institute for Machine Learning, the LIT AI Lab, and is Director of the ELLIS Unit at the Johannes Kepler University Linz. He is the founder of the company NXAI, which advances new deep learning technologies such as xLSTM. He is considered a pioneer of deep learning due to his fundamental work and historical milestones, the development of Long Short-Term Memory (LSTM) and the analysis of the Vanishing Gradient. Dr. Hochreiter's groundbreaking work on LSTM was at the core of the first large language models that later led to ChatGPT and similar models.
To ask a question remotely, visit Slido and enter #neurips2024?
In a world where rapid innovation fuels our greatest ambitions, systems and AI have found themselves in a dynamic and transformative partnership. The systems community has worked tirelessly in the background, building the foundation that enabled AI’s meteoric rise. But now, AI’s exponential progress threatens to outpace the very systems supporting it. At this critical juncture, we propose a bold “marriage”—one that allows systems and AI to co-evolve in ways that push each beyond its current boundaries.
In this keynote, we will examine the role of systems in accelerating AI advancements, the strains AI’s unprecedented growth places on current infrastructures, and the emerging ways AI can reciprocate by transforming the systems landscape. Through systems thinking and core principles, we will outline the grand challenges that arise from this union, envisioning a future where systems and AI reshape each other, charting a path forward that calls on the AI community to foster a future of symbiotic growth.
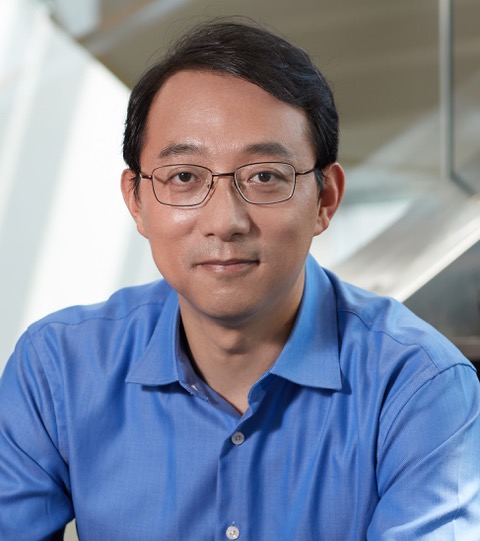
Lidong Zhou
Dr. Lidong Zhou is a Corporate Vice President at Microsoft, Chief Scientist of the Microsoft Asia Pacific R&D Group, and Managing Director of Microsoft Research Asia. With a Ph.D. in Computer Science from Cornell University, Dr. Zhou’s career at Microsoft began in 2002. His journey has spanned across Microsoft's Research labs, including the Silicon Valley lab as a researcher, the Redmond lab as a principal researcher and Research Manager of the Systems Research Group, and the Asia lab where he currently serves as the Managing Director since 2021. Dr. Zhou's expertise lies in the field of computer systems research, particularly in developing scalable, reliable, and trustworthy distributed systems, with best papers at the ACM Symposium on Operating Systems Principles (SOSP), the USENIX Symposium on Operating Systems Design and Implementation (OSDI), and the USENIX Annual Technical Conference (ATC). He has played a pivotal role in the design and development of large-scale distributed systems, which are integral to various Microsoft products and services, from search engines and big data infrastructure to cloud systems and AI infrastructure. Dr. Zhou is both an ACM Fellow and an IEEE Fellow. He has served on the editorial boards of ACM Transactions on Computer Systems, ACM Transactions on Storage, and IEEE Transactions on Computers. In 2023, he chaired the ACM Software System Award Committee and currently chairs the steering committee of SOSP. He was the general Co-Chair of SOSP 2017 and will serve as the PC Co-Chair of OSDI 2025.
To ask a question remotely, visit Slido and enter #neurips2024?
Diffusion models have revolutionized generative modeling. Conceptually, these methods define a transport mechanism from a noise distribution to a data distribution. Recent advancements have extended this framework to define transport maps between arbitrary distributions, significantly expanding the potential for unpaired data translation. However, existing methods often fail to approximate optimal transport maps, which are theoretically known to possess advantageous properties. In this talk, we will show how one can modify current methodologies to compute Schrödinger bridges—an entropy-regularized variant of dynamic optimal transport. We will demonstrate this methodology on a variety of unpaired data translation tasks.
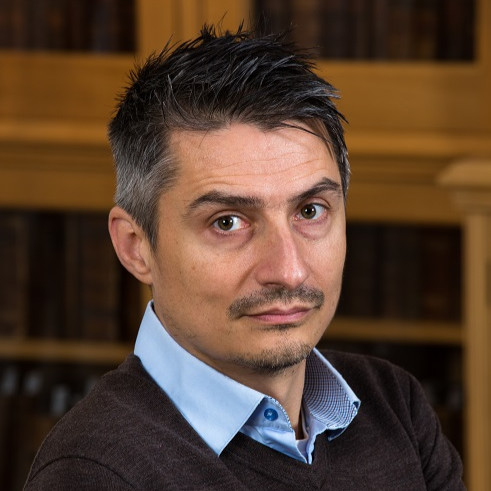
Arnaud Doucet
Arnaud Doucet obtained his PhD from University Paris-XI in 1997. He has held faculty positions at Cambridge University, the University of British Columbia, the Institute of Statistical Mathematics, and Oxford University, where he was a statutory professor in the Department of Statistics. He was an Institute Mathematical Statistics (IMS) Medallion Lecturer in 2016, was elected an IMS Fellow in 2017, and was awarded the Guy Medal in Silver in 2020 by the Royal Statistical Society for his contributions to the theory and methodology in computational statistics. Since 2023, he has been a Senior Staff Research Scientist at Google DeepMind.
To ask a question remotely, visit Slido and enter #neurips2024?
Humans learn though interaction and interact to learn. Automating highly dextreous tasks such as food handling, garment sorting, or assistive dressing relies on advances in mathematical modeling, perception, planning, control, to name a few. The advances in data-driven approaches, with the development of better simulation tools, allows for addressing these through systematic benchmarking of relevant methods. This can provide better understanding of what theoretical developments need to be made and how practical systems can be implemented and evaluated to provide flexible, scalable, and robust solutions. But are we solving the appropriate scientific problems and making the neccesarry step toward general solutions? This talk will showcase some of the challenges in developing physical interaction capabilities in robots, and overview our ongoing work on multimodal representation learning, latent space planning, learning physically-consistent reduced-order dynamics, visuomotor skill learning, and peak into our recent work on olfaction encoding.
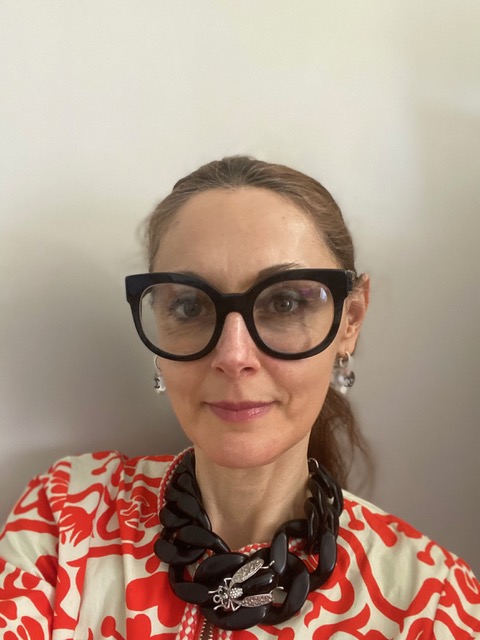
Danica Kragic
Danica Kragic is a Professor at the School of Computer Science and Communication at the Royal Institute of Technology, KTH. She received MSc in Mechanical Engineering from the Technical University of Rijeka, Croatia in 1995 and PhD in Computer Science from KTH in 2001. She has been a visiting researcher at Columbia University, Johns Hopkins University and INRIA Rennes. She is the Director of the Centre for Autonomous Systems. Danica received the 2007 IEEE Robotics and Automation Society Early Academic Career Award. She is a member of the Royal Swedish Academy of Sciences, Royal Swedish Academy of Engineering Sciences and Founding member of Young Academy of Sweden. She holds a Honorary Doctorate from the Lappeenranta University of Technology and Technical Univeristy of Rijeka. Her research is in the area of robotics, computer vision and machine learning. She received an ERC Starting and ERC Advanced grants and a Distinguished Professor Grant from the Swedish research Council. Her research is supported by the Knut and Alice Wallenberg Foundation, Swedish Foundation for Strategic Research, EU and Swedish Research Council. She is an IEEE Fellow.
To ask a question remotely, visit Slido and enter #neurips2024?
Anything is optimal given the right criteria: What are the optimal criteria as we invent the future of AI?
This talk explores this question with a series of stories including the development of affective computing,
inspired in part by how the human brain uses emotion to help signal what matters to a person.
One of these types of signals can be measured on the surface of the skin and has contributed to today’s
AI+wearable technology helping save lives. As artificial emotional intelligence abilities grow, what have
we learned about how to build optimal AI to engineer a future for people that is truly better?
Hint: It's unlikely to be achieved with scaling up today's models.